Top tips for AI readiness
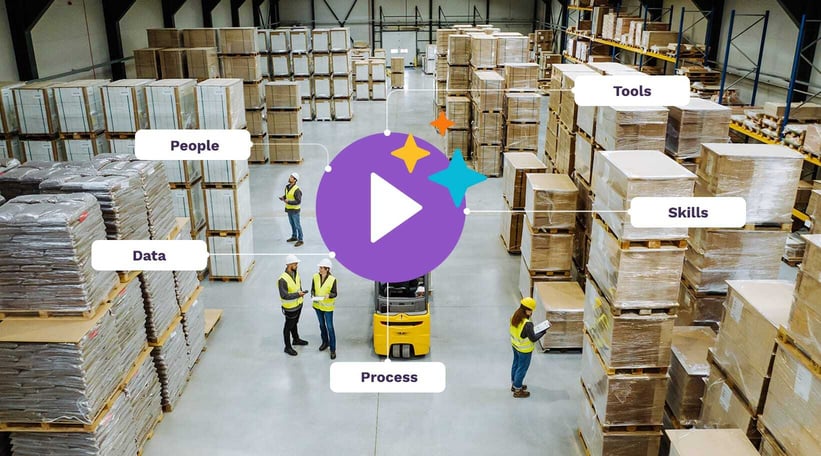
As businesses invest in AI technologies and generative AI (genAI) solutions, leaders must recognize that successful adoption is less about the tech itself and more about the readiness of your people, operations and data.
AI readiness means preparing your organization to adapt to this new era of work where automation and agentic AI become part of everyday habits. Like most innovations without proper groundwork, even the most sophisticated AI tools and machine learning models may fail to deliver the anticipated impact.
To support your company’s journey, we outline five practical strategies that foster AI preparedness from improving data quality to building a culture of trust around artificial intelligence.
AI systems rely on high-quality data feeds to function effectively. This is not a new concept in tech. If you run an ERP system and BI or FP&A software you will have strong data management or if not, you’ll have experience with all sorts of issues when the data is not reliable. AI works the same but it’s more pronounced.
1. AI starts with your data
Whether you're training generative AI tools or implementing predictive algorithms, the outcomes are only as good as the input. Poor data quality and inconsistent rules can severely limit the capabilities of advanced AI models. Clean, structured and well-managed data is essential to power any meaningful AI project.
If you haven't already, start prioritizing data governance and data management now to get AI working effectively. This includes establishing a governance framework that ensures consistent definitions, formats and access controls across divisions. Effective data governance is not just an IT responsibility, data is everyone’s business and requires buy-in from sales managers, purchasing heads and operational teams alike. A proactive AI readiness assessment should include a thorough evaluation of existing data sources, data privacy policies and how data flows through current systems. Consistent external and internal data quality controls position your business to successfully integrate artificial intelligence into your operations.
2. Are the other foundations right ?
AI implementation requires more than enthusiasm. It needs a stable foundation. If your internal systems are inconsistent or outdated, AI solutions may struggle to deliver results. Before launching full-scale AI projects, organizations need to take a hard look at their current operating model.
Do departments track performance against measurable goals? Are accountability structures in place? What is in your tech stack? These are just a sample of many questions that need to be answered honestly before moving forward with AI.
This step of the AI readiness journey is critical. AI tends to amplify existing conditions and will not correct weak processes but rather expose them. Customer success teams, AI consultants and CIOs can provide guidance to shore up these foundations and ensure your systems are ready for AI integration.
A structured approach supported by an AI readiness assessment can help identify which parts of the business are truly prepared and which may need more work. Doing this early minimizes risk and maximizes the benefits of AI implementation over time.
3. Get your people involved in AI
One of the biggest barriers to AI adoption is not technical, but human. People are naturally resistant to change, especially when it affects how they work or challenges their role. But in the context of AI, openness is vital because it is new for everyone. As new AI technologies automate routine tasks and support more complex decision-making, employees need to understand how these shifts will empower them rather than replace them.
In the exploration phase, ask your people how will the AI they are using support business goals and drive stronger results?
We recommend promoting exploration and experimentation within reason rather than blanket use of certain tools decided by management. Creating space for teams to test new applications supported by AI tools allows people to experience the benefits of AI firsthand. For example, a new user of Phocas might want to try out the natural language queries in Analytics by typing in their own question of the data and learn how the software works as they go or generate emails to customers in HubSpot.
User event sessions, hands-on video demos and internal pilot projects are all part of building a culture of learning. Encouraging questions, sharing real use cases and showcasing early wins helps employees understand the tangible benefits of AI initiatives. When people feel involved in the process, they are more likely to back change. Studies show that when employees are genuinely invested in change, the success rate can rise by up to 30%, according to McKinsey & Company.
4. Nurture champions to promote AI adoption
Successful projects need champions who are curious, motivated and excited about the potential of artificial intelligence. These internal change agents can come from any department. They might be tech-savvy operations managers, forward-thinking sales people or ambitious folk eager to improve operational efficiency.
Identifying these champions early in your AI development journey will build momentum. These individuals can pilot AI tools, provide feedback to providers or vendors, and act as trusted voices within their teams. Their enthusiasm is often contagious, helping to win over skeptics and bring together others around the broader AI strategy.
Empowering these early adopters with training and access to tools positions them as AI ambassadors.
5. Start small then build further
AI success doesn’t happen overnight. Organizations must understand that AI adoption is a staged journey, not a single event. It begins with exploration and curiosity, evolves through testing and piloting, and eventually becomes part of standard operations through trial and error.
In the exploration phase, organizations should identify specific problems AI can solve such as enhancing customer segmentation or managing rebate rules. The pilot phase then involves selecting tools, testing them in controlled environments and measuring impact. Feedback is vital here, and it should involve not only your tech team but also the end-users and stakeholders affected by changes coming from AI.
Once successful pilots prove their value, your people can begin scaling these solutions across multiple divisions. This is where broader governance comes into play, ensuring AI practices align with data privacy regulations and your overall goals.
Nothing is right straight away so let continuous improvement becomes the focus. Companies need to refine their AI models and assess the impact of AI over time and expand into new use cases. With the right support from vendors or experts, your AI strategy will start to take form.
Leading research firms like Gartner emphasize the importance of treating AI as a journey and integrating it into your broader digital transformation roadmap. Businesses that focus on change readiness, rather than rushing implementation, tend to outperform their peers in AI outcomes.
The impact of AI on business is set to be profound but without preparing your people, even the best algorithms and open source models won't deliver. Begin by improving data quality and governance, so your AI systems have the right foundation. Assess and improve your existing processes, ensuring they're ready for AI adoption. Whether you’re just starting to explore the benefits of AI or already have AI projects underway, the path to long-term success begins with readiness.

Katrina is a professional writer with a decade of experience in business and tech. She explains how data can work for business people and finance teams without all the tech jargon.
Related blog posts
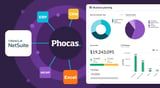
Enterprise Resource Planning (ERP) systems like NetSuite are designed to centralize your business operations, offering one platform for managing financials, customer relationships (CRM), inventory and more.
Read more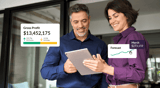
The role of the CFO is evolving rapidly, extending beyond traditional financial stewardship to encompass business partnering, operational oversight and technological innovation. A 2024 Sage study of over 1,200 global finance leaders reinforces this transformation:
Read more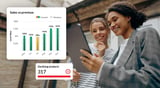
Your own data is a critical asset that can drive business growth, improve decision-making, and boost customer satisfaction. Among the various tools available, self-service reporting solves one of the most significant barriers to real-time data analysis—accessibility.
Read moreBrowse by category
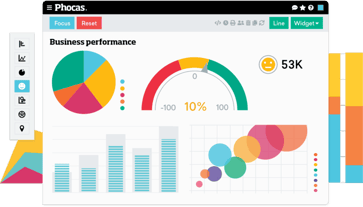
Find out how our platform gives you the visibility you need to get more done.
Get your demo today